- Teacher: Dr Surpriya M H
- Teacher: Dr Renjini D
- Teacher: Dr Zakkariya
moodle@CUSAT
Available courses
- Teacher: Dr Nimitha Aboobaker
- Teacher: Dr Santhosh Kumar G
- Teacher: Dr Mini Sekharan
- Teacher: Dr Renjini D
- Teacher: Dr Renumol V G
- Teacher: Dr Zakkariya
- Teacher: Mr Royce
- Teacher: Dr Santhosh Kumar G
- Teacher: Prof Madhu G
- Teacher: A Mujeeb
Course Description
Elementary fundamentals of linearly elastic and isotropic solid mechanics would be discussed in this course. This is a typical base course for Structural Analysis, Theory of Elasticity and Plasticity, Structural Design, Mechanics of Machinery, etc. Generally, Civil Engineering, Mechanical Engineering and Electrical Engineering graduate students would be the stake holders of this course!
Objectives
1. To introduce the basic concepts of stress, strain, displacements and elastic properties of materials.
2. To develop an affinity towards the study of internal forces in structures, like bending moment, shear force, twisting moment and axial force and their associated stresses and strains and hence to lead towards their applicability in design of structures and machine components.
3. To introduce deflection and slope of structures through elastic and statically determinate beam examples.
4. To introduce the concept of stability of structures through Euler's analysis of columns and hence to show that stability criteria is as important as strength or serviceability criteria in design of structures.
Prerequisites
1. A basic knowledge of Elementary Engineering Mechanics - Statics (Newtonian Mechanics) is expected.
2. Exposure to basic algebra, trigonometry, calculus and matrix operations.
- Teacher: Dr. Sunilkumar N.
Course Description
This is a follower course of Analysis of Structures I and II for 2012 scheme B.Tech Civil Engineering in the CUSAT. The course essentially aims at matrix based analysis of structures and introduction to the finite element method (elementary level, only). Introduction to computer methods of analysis of structures can also be a part of discussion.
Course Objectives
1. To introduce the basics of flexibility and stiffness and hence pave way to systematic methods for structural analysis.
2. To introduce the concept of writing the linear algebraic equations generated as part of the analysis of structures through the method of consistent deformation or the slope-deflection method in matrix form and solving them after applying the boundary conditions.
3. Introduction of the direct stiffness method to throw light to the need of computer implementation of the method and give a step-by-step procedure for the same.
4. To eradicate an expected fearful approach of a student of civil engineering towards the most versatile numerical tool in engineering, the finite element method through introduction of the same via. the stiffness method.
Prerequisites
1. Exposure to statically indeterminate structural analysis through consistent deformation and slope deflection methods.
2. Basics of strength of materials.
3. A good level of understanding of determination of deflection and slope of basic structures (beams and portal frames).
4. Sound mathematical ability in linear algebra, fundamental calculus and basic trigonometry.
- Teacher: Dr. Sunilkumar N.
Course Description
The fundamentals of the most versatile numerical tool in engineering and science, the Finite Element Method (FEM), limitations of applicability of the FEM, introduction to the open world of a class of methods, named as the Mesh-free methods (M-Free) and description of one of such methods would be attempted in this course.
Course Objectives
1. Introduce the Finite Element Method from the scratch through solid mechanics applications.
2. Motivate the students to generate an affinity towards assimilating the fundamentals of the FEM before attempting any computer program of which the FEM is a black box.
3. Illustrate the FEM (in 1D and 2D) through certain typical benchmark examples from solid mechanics and numerical procedure for integration, solution of equations (linear, non-linear and eigen value problems).
4. Throw light on the limitations of the applicability of the mesh-based methods and the remedies adopted to overcome the same, in the literature.
5. Introduce the mesh free concept and illustrate the same through one of the integral equation representation mesh-free methods, the Reproducing Kernel Particle Method (RKPM).
Prerequisites
1. Exposure to linear solid mechanics (equations of equilibrium, compatibility, boundary conditions, applications, etc.).
2. Exposure to linear algebra (matrices and their operations), calculus and partial differential equations.
- Teacher: Dr. Sunilkumar N.
- Teacher: Dr Josephkutty Jacob
Financial Management for Engineers
- Teacher: Dr Mathew Cherian
- Teacher: Dr. Job P. Antony
Computer Aided Design and Management
- Teacher: Dr Mathew Cherian
- Teacher: Dr Santhosh Kumar G
- Teacher: Prof Madhu Gopal
- Teacher: Prof Madhu G
- Teacher: Kulshrestha Deepti
- Teacher: Admin User
- Teacher: Dr Suja Devipriya
- Teacher: Dr Santhosh Kumar G
- Teacher: Dr. Madhu S. Nair
- Teacher: Dr Santhosh Kumar G
- Teacher: Dr Praneetha P
- Teacher: R Kala
- Teacher: Dr Suja Haridas
- Teacher: E Manoj
- Teacher: Dr Sebastian Nybin Remello
- Teacher: Dr Suja Haridas
- Teacher: R Kala
- Teacher: Dr Manoj N
- Teacher: R Kala
- Teacher: Dr Sabura P M
- Teacher: Dr V Sivanandan Achari
- Teacher: Usha K
- Teacher: Asha A S
- Teacher: Anoop K.K.
- Teacher: Senoy Thomas
- Teacher: Dr Santhosh Kumar G
- Teacher: Harigovind P C
Fundamentals of Corporate Laws
Course Objective
This course is designed to enable the students in understanding the basic laws governing companies.
Expected Outcome of the course:
On completion of the course the student will be able to
- Appraise the legal framework governing companies
- Understand the business models available for doing business, the advantages and disadvantages of each business model
- Understand the principles of governance of companies
- Understand different methods of raising capital and sources of corporate finance
- Appraise the life cycle of companies and the structural changes.
- Teacher: Dr Santhosh Kumar G
- Teacher: Dr. Madhu S. Nair
- Teacher: Preetha S
- Teacher: S Sreejith
Interdisciplinary Elective
CONSUMER PROTECTION LAWS
Total Credits: 4
Mode of Offering: MOOC Course
Course Teacher: Dr. Aneesh V. Pillai, Assistant Professor, School of Legal Studies,
Course Code: 22-405-0406
Course Objective:
This course is intended to provide an idea about the scope and application of consumer protection laws in India. This further discusses the various quality standards and marks for the protection of consumers as well as the issue of defect and deficiency in goods and services. This course also provides an idea about the mechanisms for the enforcement of consumer rights in India. The course intends to cover contemporary judicial and legal developments in this area.
Learning Outcomes:
On successful completion of this course, the learner will be able:
1) To understand about various consumer rights, the laws dealing with such rights and to act as a responsible consumer in the market
2) To understand the unfair practices in the market and advice about the same to his friends and relatives
3) To pursue remedies not only for him but on behalf of others against defect or deficiency
Course Content:
Module I: Introduction
Consumer: Meaning and Scope - Rights of Consumers – History of Consumer Protection - Consumer Protection Laws – General Laws – Specific Laws – Voluntary Mechanisms
Module II: Consumer Protection Laws
Civil Laws – Criminal Laws - Consumer Protection Act, 2019 – Key Features – New Definitions – Central Consumer Protection Authority – Mediation - Other important Features
Module III: Defects in Goods
Goods - Consumer of Goods – Defects – Remedies – Judicial Decisions - Unsafe and Hazardous Products - Product Liability - Quality Certification Marks and Standards
Module IV: Deficiency in Service
Service - Deficiency in Service – Remedies – Deficiency in Different Services - Unsafe and Hazardous Service - Service Liability – Medical Service & Consumer – Advertisements
Module V: Enforcement of Consumer Rights
Agencies under Consumer Protection Act - Central Consumer Protection Authority – Consumer Protection Councils - Consumer Commissions - Jurisdiction, Functions and Powers - Procedure – Enforcement of Orders – Mediation
References:
1. Dr. Avtar Singh, Consumer Protection Laws, EBC, Lucknow, 2018
2. G. B. Reddy, Law of Consumer Protection, Hyderabad: Gogia Law Agency, 2014
3. Mamta Rao, Public Utility Services under the Consumer Protection Act, Deep and Deep Publications, New Delhi, 1998
4. V N Viswanathan, Consumer Rights in Service Sector, D. K. Agencies, New Delhi, 2008.
5. Rifat Jan, Consumerism and Legal Protection of Consumers, Deep & Deep Publishers, New Delhi, 2007.
6. MM Nabi, et al, Consumer Rights and Protection in India, New Century Publications, 2015
7. D P Wadhwa & N L Rajah, The Law of Consumer Protection (Set of 2 Volumes), Lexis Nexis, 2017
8. Dr. H. K. Saharay, Text Book on Consumer Protection Law, Universal Law Publishing Co, 2017
9. P K Dutta, Consumerism and Consumer Protection in India: Law and Practice, Himalaya Publishing Co, 2015.
- Teacher: Dr Santhosh Kumar G
- Teacher: Dr. Aneesh V. Pillai
- Teacher: Harigovind P C
- Teacher: Dr. Aneesh V. Pillai
- Teacher: S Sreejith
- Introduction and the need for intellectual property right (IPR) - Kinds of Intellectual Property Rights: Patent, Copyright, Trade Mark, Design, Geographical Indication, Plant Varieties and Layout Design – Genetic Resources and Traditional Knowledge – Trade Secret - IPR in India : Genesis and development – IPR in abroad - Major International Instruments concerning Intellectual Property Rights: ( 10 Hours)
- Concept of Trademarks - Different kinds of marks (brand names, logos, signatures, symbols, well known marks, certification marks and service marks) - Non Registrable Trademarks - Registration of Trademarks - Rights of holder and assignment and licensing of marks - Infringement, Remedies & Penalties - Trademarks registry and appellate board Trade secrets – Geographical indications- Traditional Knowledge – (10 Hours)
- Patents - Elements of Patentability: Novelty , Non Obviousness (Inventive Steps), Industrial Application - Non - Patentable Subject Matter - Registration Procedure, Rights and Duties of Patentee, Assignment and licence , Restoration of lapsed Patents, Surrender and Revocation of Patents, Infringement, Remedies & Penalties - Patent office and Appellate Board (8 Hours)
- Nature of Copyright - Subject matter of copyright: original literary, dramatic, musical, artistic works; cinematograph films and sound recordings - Registration Procedure, Term of protection, Ownership of copyright, Assignment and licence of copyright - Infringement, Remedies & Penalties – Related Rights - Distinction between related rights and copyrights-----( 10 Hours)
- Design meaning and concept of novel and original - Procedure for registration, effect of registration and term of protection Geographical Indication (GI) Geographical indication: meaning, and difference between GI and trademarks - Procedure for registration, effect of registration and term of protection Plant Variety Protection Plant variety protection: meaning and benefit sharing and farmers’ rights – Procedure for registration, effect of registration and term of protection Layout Design Protection Layout Design protection: meaning – Procedure for registration, effect of registration and term of protection
- IP Management - Concept of IP Management - Intellectual Property and Marketing - IP valuation methods (Theory) India`s New National IP Policy, 2016 – Govt. of India step towards promoting IPR – Govt. Schemes in IPR – Career Opportunities in IP - IPR in current scenario with case studies ------------------( 7 Hours)
- Teacher: Dr. Anson C J
- Teacher: Dr. Anson C J
- Teacher: Dr. Anson C J
- Teacher: Dr Santhosh Kumar G
- Teacher: Dr Mohammed Hata
- Teacher: Dr Santhosh Kumar G
- Teacher: Dr Ancy V P
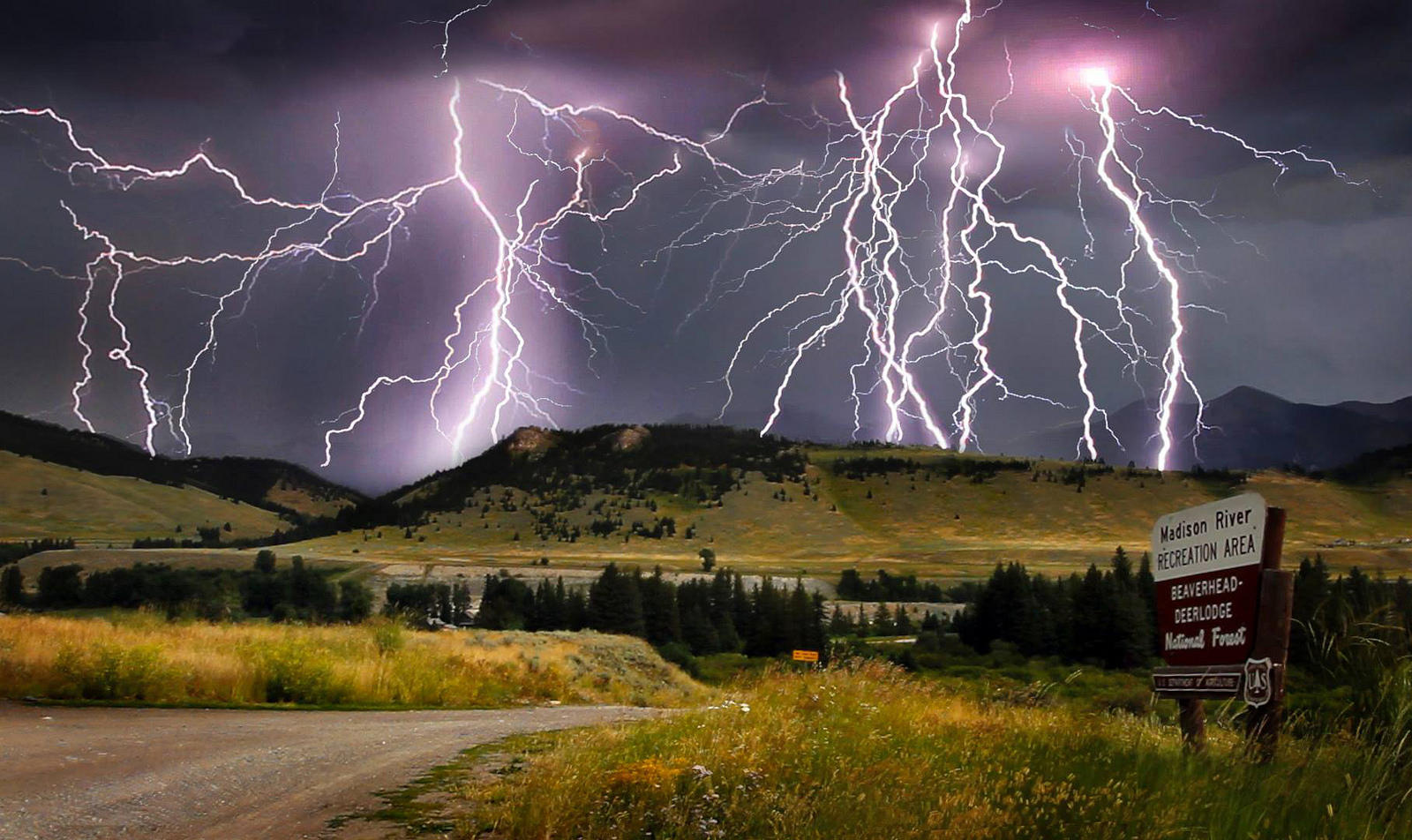
Cloud physics is the study of the physical processes that lead to the formation, growth and precipitation of atmospheric clouds. These aerosols are found in the troposphere, stratosphere, and mesosphere, which collectively make up the greatest part of the homosphere. Clouds consist of microscopic droplets of liquid water (warm clouds), tiny crystals of ice (cold clouds), or both (mixed phase clouds). Cloud droplets initially form by the condensation of water vapor onto condensation nuclei when the supersaturation of air exceeds a critical value according to Köhler theory. Cloud condensation nuclei are necessary for cloud droplets formation because of the Kelvin effect, which describes the change in saturation vapor pressure due to a curved surface. At small radii, the amount of supersaturation needed for condensation to occur is so large, that it does not happen naturally.
Atmospheric electricity refers to electricity that exists in the atmosphere as a result of natural phenomenon. The primary natural source of atmospheric electricity is thunderstorm activity, with lightning being the most spectacular and obvious manifestation of this electricity. However, there are other aspects of atmospheric electricity that cannot be seen visibly, but are helping scientist understand changes in the earth's climate.
After completing the course the student will be able to
- Able to identify different types of clouds
- Formation of cloud droplets and clouds
- Understand the theory of Droplet Growth
- Understand the formation and growth of Ice crystals
- Understand the precipitation process
- Acquire the knowledge of Severe storm and Hail formation
- Understand weather modifications
- Acquire the knowledge of thunderstorm formation and lightening
- Understand about the atmospheric electricity
- Acquire the knowledge about the precautions during the thunder and lightning.
- [ ALL TYPES OF CLOUDS]
- Teacher: Dr Madhu V
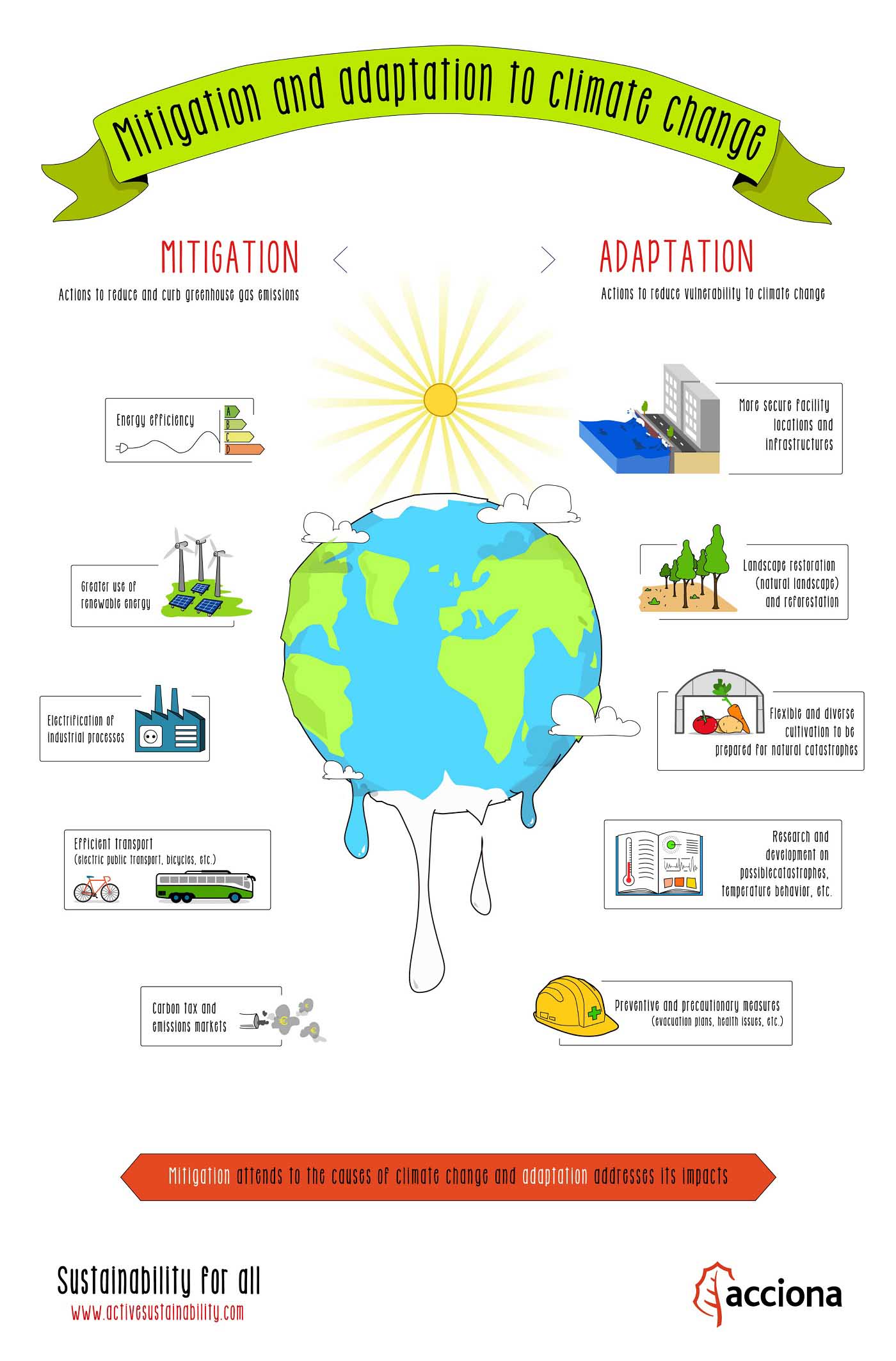
When it comes to tackling climate change to prevent the impacts it causes in the different systems of the planet, the human being applies two types of measures: mitigation and adaptation.
Mitigation measures are those actions that are taken to reduce and curb greenhouse gas emissions, while adaptation measures are based on reducing vulnerability to the effects of climate change.
Mitigation, therefore, attends to the causes of climate change, while adaptation addresses its impacts.
How to mitigate climate change?
These are some of the mitigation measures that can be taken to avoid the increase of pollutant emissions:
- Practice Energy efficiency
- Greater use of renewable energy
- Electrification of industrial processes
- Efficient means of transport implementation: electric public transport, bicycle, shared cars ...
- Carbon tax and emissions markets
Adaptation to climate change:
In terms of adaptation measures, there are several actions that help reducing vulnerability to the consequences of climate change:
- More secure facility locations and infrastructures
- Landscape restoration (natural landscape) and reforestation
- Flexible and diverse cultivation to be prepared for natural catastrophes
- Research and development on possible catastrophes, temperature behavior, etc.
- Preventive and precautionary measures (evacuation plans, health issues, etc.)
In this infographic you can learn what are the measures of adaptation and mitigation to climate change
https://www.youtube.com/watch?v=QUegpxhMuQ4
- Teacher: Dr Madhu V
This course is aimed to achieve following objectives....
-
Describe different types of weather systems
-
Analyze characteristic features of dominant weather systems over Indian region
-
Interpret and classify different scale of the weather features and forecasting strategies
-
Assess challenges and limitations of various forecast process
-
Predict weather systems at different space-time domains using synoptic and NWP methods
-
Devise and develop forecast process
-
Create real time forecast
- Teacher: Dr Abhilash
After completing the course, students will be able to
-
Understand coding-decoding.
-
Analyse different charts.
-
Examine dominant weather systems using synoptic charts.
-
Reading diffferent NWP charts.
-
Dignosis and prognosis of weather systems using synoptic/NWP charts.
- Teacher: Dr Abhilash
The middle atmosphere (stratosphere and mesosphere) is the region of the atmosphere between about 16 and 100 km altitude. Studies of dynamical and chemical processes in this region have greatly expanded in recent years owing to the impact of human activities on the stratospheric ozone layer, and the coupling between stratospheric changes and surface climate. A primary area of emphasis is study of the dynamical interactions between the troposphere and the stratosphere, including the transfer of momentum and trace constituents across the tropopause. This effort requires understanding of the influence of both large- and small-scale wave motions on the momentum balance and mass circulation of the middle atmosphere.
The prevailing winds in the atmosphere near the equator above about 17 km are known to undergo a slightly irregular oscillation from strong westerlies to strong easterlies roughly every other year. This Quasi-biennial Oscillation (QBO) has been observed to dominate the winds in this region of the atmosphere for at least the last 6 decades and is characterized by a downward propagation of the westerly and easterly jets that form.
El Niño and La Niña are opposite phases of what is known as the El Niño-Southern Oscillation (ENSO) cycle. The ENSO cycle is a scientific term that describes the fluctuations in temperature between the ocean and atmosphere in the east-central Equatorial Pacific (approximately between the International Date Line and 120 degrees West).
La Niña is sometimes referred to as the cold phase of ENSO and El Niño as the warm phase of ENSO. These deviations from normal surface temperatures can have large-scale impacts not only on ocean processes, but also on global weather and climate.
El Niño and La Niña episodes typically last nine to 12 months, but some prolonged events may last for years. While their frequency can be quite irregular, El Niño and La Niña events occur on average every two to seven years. Typically, El Niño occurs more frequently than La Niña.
- Teacher: Dr Madhu V
Recombinant DNA technology is a 3 credit core course for the M.Sc. Semester III Biotechnology program. This is an advanced course dealing with the tools and techniques involved in manipulating DNA. The various modules elaborate the different enzymes, the types of vectors used, the expression systems, the heterologous host systems used as well as the various cloning strategies and the processes involved therein. In addition techniques such as PCR, blotting, site directed mutagenesis, gene transfer and various screening strategies are included.
After completing the course the student will be able to
-
Elaborate the different enzymes, vectors, as well as cloning strategies
-
Apply the different enzymes used in rDNA technology
-
Use different types of vectors for cloning
-
Produce a genomic DNA library and screening for recombinants
-
Construct a probe and do blotting techniques
-
Apply site directed mutagenesis technique
-
Employ different types of PCR techniques for gene amplification and clone the amplicon
-
Demonstrate heterologous gene expression
-
Compare various genome editing tools
- Teacher: Dr Sarita G Bhat
thisnnn
- Teacher: Dr Sarita G Bhat
- Teacher: Dr. Yoosaf K
- Teacher: R Kala
- Teacher: R Kala
- Teacher: Dr Manoj N
- Teacher: Dr Leena R
- Teacher: E Manoj
- Teacher: Dr Sebastian Nybin Remello
- Teacher: Dr Suja Haridas
- Teacher: E Manoj
- Teacher: Dr Leena R
- Teacher: Dr P V Mohan
- Teacher: R Kala
- Teacher: Dr Sabura P M
- Teacher: Dr Sebastian Nybin Remello
M. Sc. Chemistry, Semester II
CHE 2204 Organic Chemistry-III
(Reagents and Synthesis)
- Teacher: Sindhu Mathai
- Teacher: Dr Sebastian Nybin Remello
- Teacher: Dr Suja Haridas
- Teacher: Dr Manoj N
- Teacher: Sindhu Mathai
- Teacher: Dr Sabura P M
- Teacher: Susmita De
- Teacher: Dr K Sreekumar
- Teacher: Dr Sebastian Nybin Remello
- Teacher: Dr Suja Haridas
- Teacher: Susmita De
- Teacher: R Kala
- Teacher: Dr Leena R
- Teacher: Sindhu Mathai
- Teacher: Dr Sebastian Nybin Remello
- Teacher: Dr Sebastian Nybin Remello
- Teacher: Dr Suja Haridas
- Teacher: Sindhu Mathai
- Teacher: Prathapan S
- Teacher: Dr P V Mohan
- Teacher: Dr Sebastian Nybin Remello
- Teacher: Dr Suja Haridas
Total Number of Credits - 3
- Core Course
- 3 hours of lecture and 1 hour tutorial
- Total 48 hours of contact sessions
Continuous evaluation - 50 Marks (30 + 10 + 10)
- 30 Marks from two online internal exams on the moodle platform having 15 Marks each
- 10 Marks for Assignment in the form of quizzes during live online classes, quizzes as part of recorded sessions
- 10 Marks for an End semester online Viva - Voce examination
End semester examination - 50 Marks
Mode of End Semester examination will be communicated to you by the university depending on the pandemic situation
- Teacher: Dr Manoj N
- Teacher: R Kala
- Teacher: Dr Manoj N
- Teacher: R Kala
- Teacher: Dr Manoj N
- Teacher: Susmita De
- Teacher: R Kala
- Teacher: Dr Leena R
- Teacher: Dr Sebastian Nybin Remello
- Teacher: Dr Suja Haridas
- Teacher: Dr K Sreekumar
- Teacher: Dr Leena R
- Teacher: E Manoj
- Teacher: Dr Sebastian Nybin Remello
- Teacher: Dr Suja Haridas
- Teacher: A A Ambily
- Teacher: A A Ambily
- Teacher: Dr Aldrin Antony
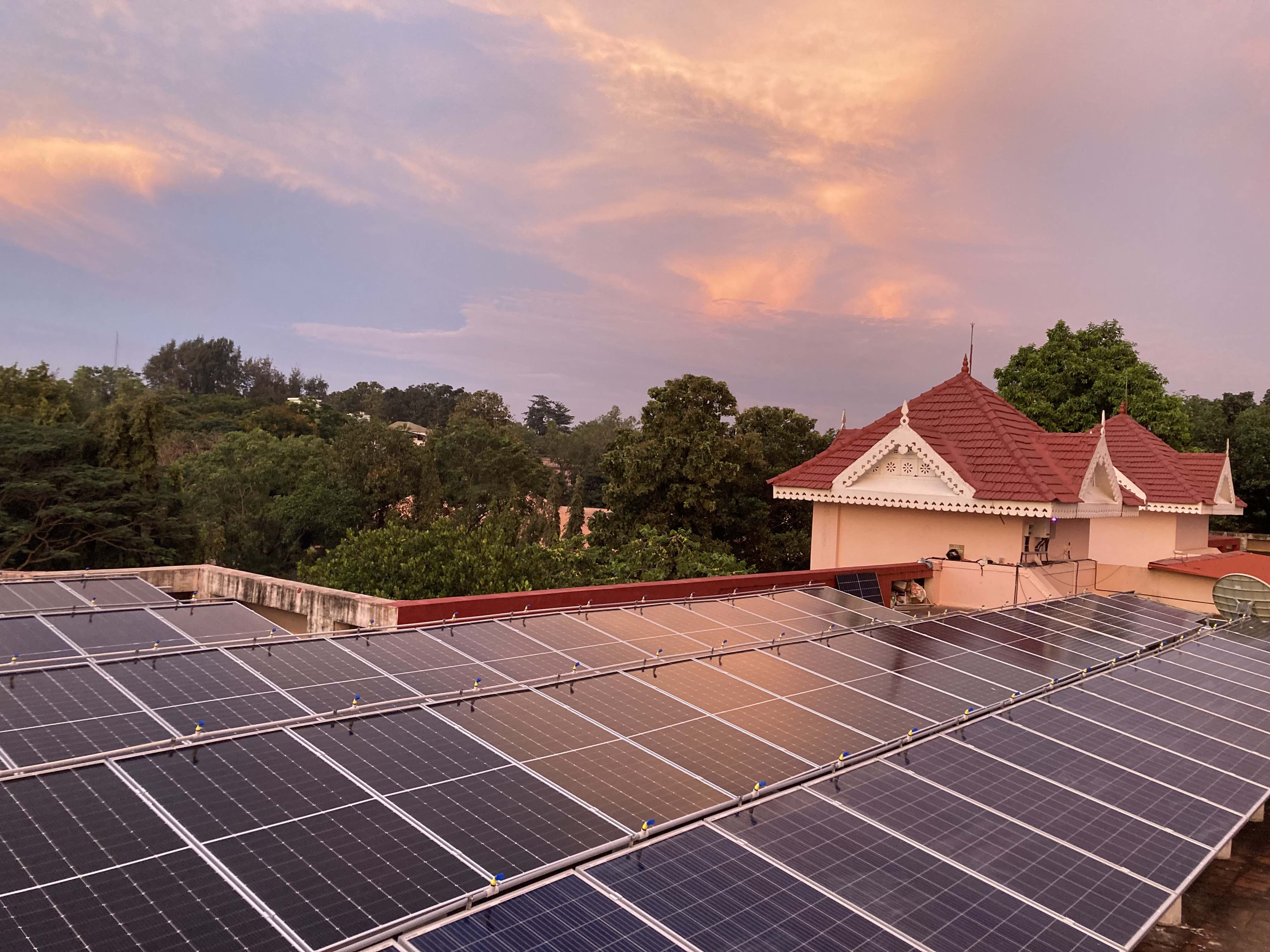
Interdepartmental Elective offered from Centre of Excellence in Advanced Materials.
Course Co-ordinator: Dr. Aldrin Antony, Department of Physics
- Teacher: Dr Aldrin Antony
- Teacher: Asha A S
- Teacher: Dr Aldrin Antony
- Teacher: Jayaraj MK
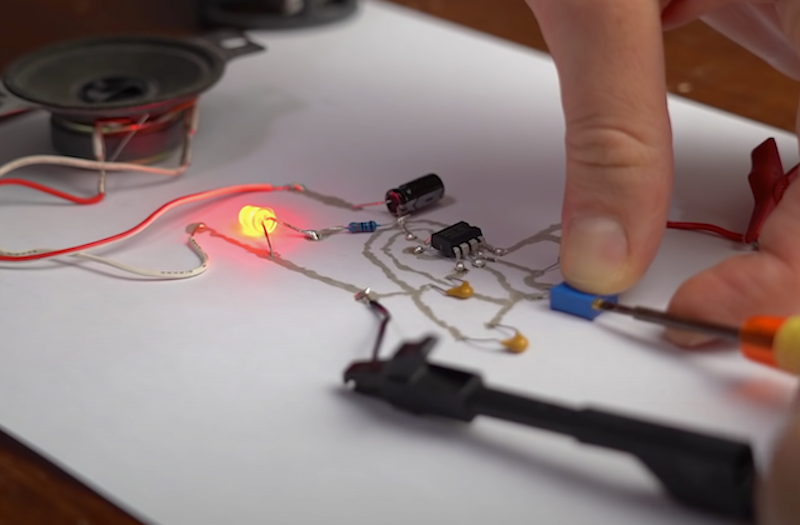
This is a basic course in Electronics which will give an idea about different semiconductor devices and digital electronics.
- Teacher: Dr Aldrin Antony
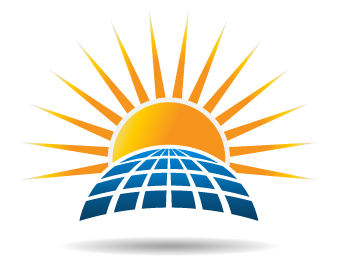
- Teacher: Dr Aldrin Antony
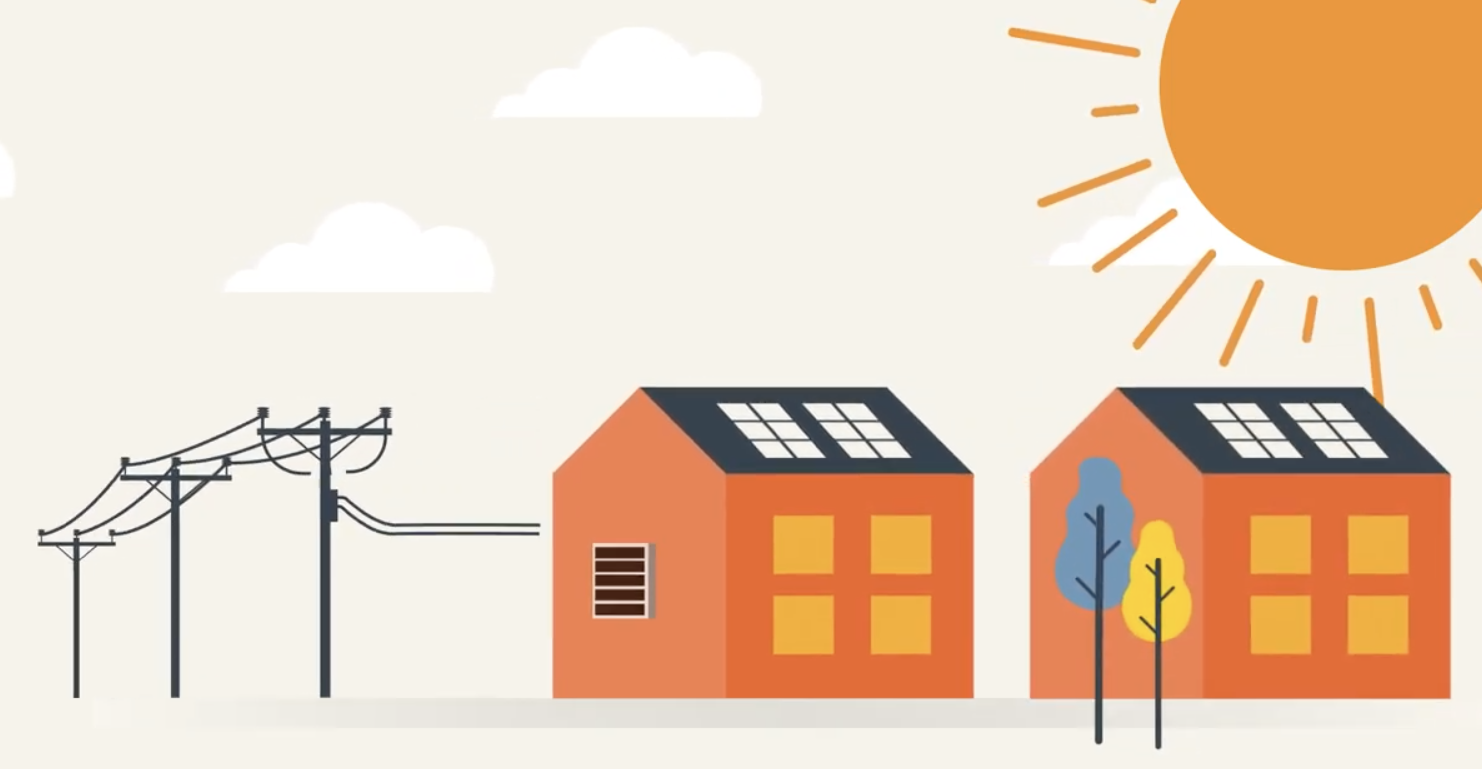
Course Objectives
The objective of the course is to develop a general understanding of the need for clean energy sources and the potential and application of photovoltaic (PV) technology to generate power. The course will give an insight in the fabrication of the solar cells in laboratory and industrial scale, module fabrication and power generation using PV in o↵ grid and grid connected systems.
Course Outcomes
After the successful completion of the course the students will be able to confidently:
-
Understand PV based electricity generation
-
Differentiate the manufacturing and performance differences between different c- Si wafer technologies and between ? c-Si and thin film PV technologies
-
Calculate the power and energy produced by a solar module
-
Explain the differences and design aspects of off-grid and on-grid PV systems.
-
Basic knowledge to use PVSyst
-
Explain various current and futuristic applications of PV
- Teacher: Dr Aldrin Antony
- Teacher: Dr Aldrin Antony
- Teacher: Dr Aldrin Antony
- Teacher: Titus K Mathew
- Teacher: Jayaraj MK